To ensure equitable health care access and delivery in the future, artificial intelligence designers and decision makers will need to follow bias avoidance best practices.
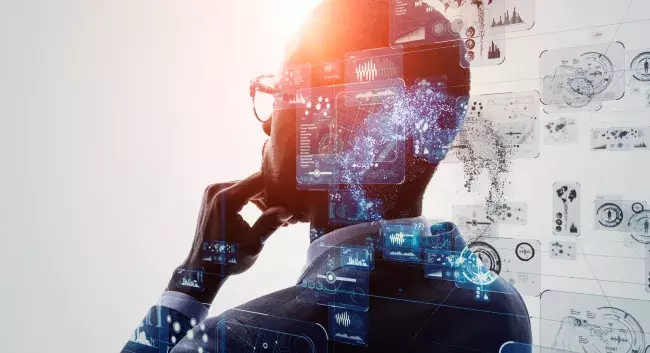
Addressing algorithmic bias and the perpetuation of health inequities: An AI bias aware framework
You would not want to live in a world where the medical app you use discriminates against you.
In 2021, the CDC acknowledged systemic racism as a serious threat to public health. However, algorithms that use artificial intelligence/machine learning (AI/ML) approaches are often prone to bias, which can be introduced unknowingly. For example, one algorithm that used health care spending as a proxy for illness ended up prioritizing affluent white patients. In addition, dermatological models that lack sufficient skin color representation could exacerbate disparities in cancer diagnoses. And language models can absorb harmful associations in the context of medical notes. In an age where the digital transformation of health care has become more widespread, algorithmic bias may perpetuate and compound health inequities.
This leads to two urgent questions: what algorithmic biases exist in health care and what can we do about them?
A recent research paper co-authored by CDHAI researchers together with colleagues from Accenture, the World Bank, and the University of Maryland describes a four-level analytical process for building and deploying health care solutions that incorporate AI/ML, addressing the problem scope, data, model, and decision. The paper highlights the sources of bias and methods for bias mitigation for each of the various levels of the process. The authors also offer recommendations for furthering the pursuit of algorithmic fairness in different health care contexts and scenarios; these recommendations include developing and using representative datasets, debiasing, and the use of race corrections.
To ensure equitable health care access and delivery in the future, AI designers and decision makers will need to follow bias avoidance best practices. The framework in this paper is crucial to future implementation and application practices that will facilitate equitable patient care and health outcomes that are free of racial disparities and biases. CDHAI promotes and encourages the adoption of bias mitigation practices as a norm or standard in future development of health care AI/ML.