Researchers call for responsible AI frameworks to ensure new health care technologies do not worsen or create new health disparities and inequities.
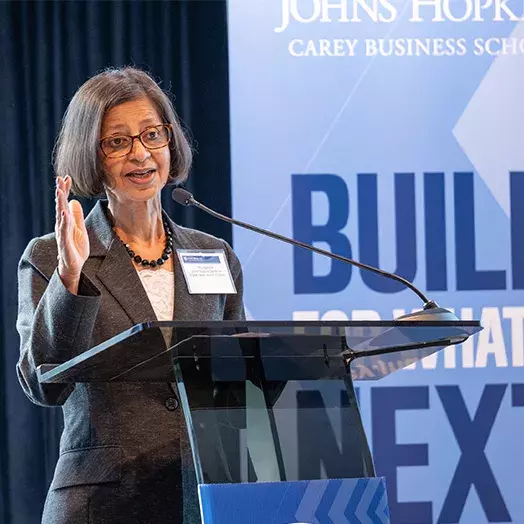
Researchers warn of the potential for “digital redlining” as AI permeates health care
“First, do no harm” is a guiding principle for physicians and health care providers, but what are the standards for emerging artificial intelligence algorithms and digital technologies?
“Many of us don't even know when an AI is making a decision that affects us,” said Wm. Polk Carey Distinguished Professor Ritu Agarwal, who co-leads the Johns Hopkins Carey Business School Center for Digital Health and Artificial Intelligence. “So, in such an algorithm-driven world, we should be worrying about the impact of this AI on people. And especially in the health care sector, that is of immense consequence What is the impact of this for each and every person on this planet?”
With CDHAI Co-director Professor Gordon Gao and colleagues from multiple academic units across Johns Hopkins University, Agarwal convened the inaugural Responsible AI for Health Care Symposium to explore research and policies to ensure new health care technologies do not worsen or create new health disparities and inequities.
We operate, Agarwal said, in a society with longstanding and deep-rooted health disparities and inequities. According to an analysis by the Kaiser Family Foundation, Black and American Indian or Alaska Native people fare worse than their white counterparts across numerous health measures including infant mortality, pregnancy-related mortality, diabetes mortality, and cancer mortality despite years of attention and attempts to address the issues.
What to Read Next
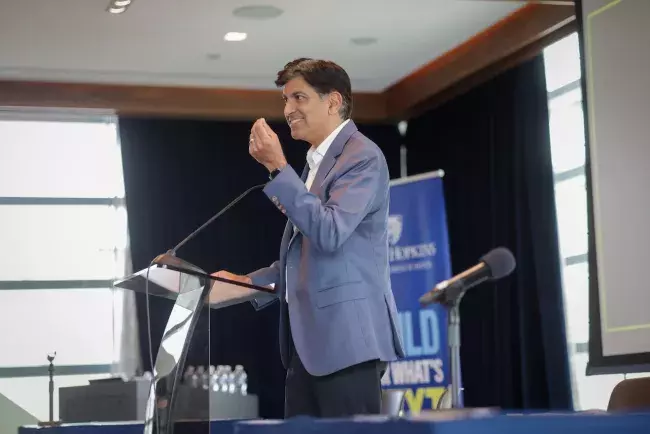
business of health
Can AI and digital health solve health care's biggest challenges? Insights from the Conference on Health IT and AnalyticsDigital redlining
RAIHS keynote speaker Maia Hightower is a physician and a leading voice on the issue of health equity and digital technology. She is also CEO and co-founder of a startup called Equity AI, which helps clinical teams achieve health equity through responsible AI and machine learning operations and is a former executive vice president and chief digital and technology officer at the University of Chicago Medicine. In her address, Hightower outlined many of the inherent challenges and barriers to bringing AI to the health care sector.
“There is nothing in health care that you can say consistently is systematized,” said Hightower. “If you go to Starbucks down the street or the Starbucks in China, or you go to the Starbucks in Japan there's a consistency. You know you are in Starbucks. That consistency, the standardization does not exist in health care, and that complexity makes AI translation so very challenging.”
Hightower warns that the nature of AI technologies and the complexities within the health care system could lead to what she calls “digital redlining,” which could make these new technologies inaccessible or unobtainable for traditionally marginalized groups. “Redlining” refers to discriminatory housing policies historically applied in many U.S. cities to prohibit Black people and other groups from purchasing homes in certain areas.
“Often when it comes to digital redlining, we do it implicitly, ignorantly,” explained Hightower.
As an example, she noted that early speech recognition technologies used by physicians, such as Dragon, worked well for native English speakers while leaving physicians with accents frustrated. Similarly, the most widely used digital patient portals only use one or two languages and are only accessible to patients able to own computers or smartphones. In another example, she cited research regarding an AI algorithm that used health care spending as a predictor for diabetes risk. “It turns out African American populations systematically spend less while being equally sick. So, the developers just perpetuated an [existing] inequity and disparity in the model at scale across hundreds of health systems,” Hightower said.
When trying to address health disparities, Hightower explains, bias can also creep into the quantitative fairness metrics used to assess and mitigate bias in machine learning models.
“It can be very challenging for an analyst or for a data scientist to choose which fairness metrics to prioritize, especially when you have some fairness metrics that prioritize accuracy, some that prioritize equitable distribution of resources, and others that prioritize harm avoidance,” she said.
Next steps
Hightower notes that the potential bias exists at all levels of the AI life cycle, from data creation to model development to real-world implementation.
“But the good news is there are really well-established, researched, evidence-based methods to mitigate biases across the lifecycle.”
According to Hightower, AI quality assurance and management starts with strong capabilities around AI governance with an ability to audit and monitor models over time, and digital solutions always have patient outcomes and communities in mind. She adds that it is essential to review models from the technical perspective of how they operate, as well as understand how they are utilized by practitioners.
Some policymakers are already beginning to act. In August, California legislators passed SB 1047, a measure that would require businesses using AI to follow safeguards and hold companies liable for any harm their AI algorithms cause. The measure is awaiting the governor’s signature to be enacted. Earlier this year, Colorado passed laws that limit the development and usage of AI systems that make "consequential decisions," including decisions about health care.
In addition to Hightower, RAIHS featured speakers Emma Pierson, Andrew H., and Ann R. Tisch Assistant Professor at Cornell Tech who discussed health equity challenges of data, and Cynthia Rudin, Gilbert, Louis, and Edward Lehrman Distinguished Professor of Computer Science who spoke about the benefits of interpretable neural networks over “black boxes” in radiology.
The symposium also included three panels, the first of which centered on the discussion of metrics that can be used to measure the fairness, risk, and trustworthiness of AI models. Panelists included Brad Malin (Vanderbilt), Anjana Susarla (Michigan State), Arya Farahi (University of Texas at Austin), and Jun Deng (Yale). The second panel focused on the implementation of AI in clinical settings and featured panelists Yiye Zhang (Weill Cornell), Roy Adams (Johns Hopkins), and Abu Mosa (Missouri). The final panel emphasized the need to educate clinicians and the next generation of scholars, featuring clinicians Keila Lopez (Baylor College of Medicine) and Jasjit Singh Ahluwalia (Brown) and academic researchers Dongsong Zhang (UNC Charlott) and Xinghua Lu (University of Pittsburgh).
RAIHS was supported by the Johns Hopkins Nexus Awards, a university-wide initiative that supports convening, research, and teaching at the Johns Hopkins Bloomberg Center in Washington, D.C. The awards recognize a wide range of interdisciplinary projects and programs.