Professors Ritu Agarwal’s and Gordon Gao’s research demonstrate a new way to fight fraud in health care reviews using large language models.

Identifying fake physician reviews: The case for large language models
When shopping online for a new health care provider, many of us rely on patient reviews to guide our decision-making. Given the influence these reviews can have, it’s perhaps not surprising that in medicine—as in many industries—some bad actors post fraudulent reviews in an effort to sway patient choice.
“Given the potentially severe and adverse consequences for individuals’ health outcomes, fake reviews in health care are particularly risky, prompting concern among consumer groups and regulatory bodies,” notes Ritu Agarwal, the Wm. Polk Carey Distinguished Professor of Information Systems and Health at Johns Hopkins Carey Business School.
To distinguish real physician reviews from fake ones, Agarwal, Carey Business School Professor Gordon Gao, and colleagues from Simon Fraser University tapped into the power of large language models, specifically the generative pre-trained transformer models used by GPT-3 and GPT-4, in a recent study, “Catch Me If You Can: Identifying Fraudulent Physician Reviews with Large Language Models Using Generative Pre-Trained Transformers.”
“Our findings reveal significantly superior performance of GPT-3 over traditional machine learning in this context,” says Gao, who is co-director along with Agarwal of the Center for Digital Health and Artificial Intelligence, or CDHAI. “We were able to separate the wheat from the chaff, demonstrating that we now have a new way to fight fraud in health care reviews.”
Democratizing machine learning
Gao and Agarwal note in their paper that the linguistic traits that distinguish a fraudulent review from an authentic one “can be very subtle.” To illustrate their point, the researchers include a table with examples of 10 real online doctor reviews and invite readers to discern which are fake.
The researchers note in their paper that they also ran a simple lab experiment on a crowdsourced platform asking people to rate a random sample of reviews. “The results suggested that humans were more likely to label genuine reviews as fake and fake reviews as genuine than to label the classes correctly,” they write.
Given the unreliability of human analysis, researchers have turned to computers. “But up to this point, the tools on-hand have not proven altogether satisfactory in identifying fraudulent reviews, and they have been even less capable of identifying the characteristics that distinguish fake from genuine reviews,” notes Agarwal.
She and Gao point out that previous analyses have utilized machine learning and natural language processing, which focus on a set of specific features such as specific words. By employing GPT-4, the newest member in the GPT family, the researchers were able to tease out key dimensions along which fake and real reviews differ. That’s because the GPT-4 approach “considers the entire word sequence in the review text instead of focusing on a set of linguistic features like specific words,” they write.
In “Catch Me If You Can,” the Carey researchers, alongside Ashwarya Deep Shukla, Laksh Agarwal, and Jie Mein Goh, tested their approach on a dataset of 38,048 doctor reviews obtained from one of the largest doctor platforms in India.
“We really weren’t sure what to expect before we tried GPT,” says Gao. “It was quite remarkable to see how well we were able to label the fake reviews.”
An additional benefit of using GPT-4, the researchers say, is that it requires a smaller training sample than traditional models and is even effective using a “cold start context,” such as when there are no previous reviews of a doctor.
Companies often struggle to obtain sufficiently large, labeled datasets, so the ability to achieve high performance with small datasets, they point out, “represents a crucial step towards democratizing machine learning and enabling more companies to benefit from its applications at a modest cost.”
Key differences in writing style
At the end of their paper, Gao and Agarwal include a table that summarizes key differences they found between genuine and fake physician reviews.
What to Read Next
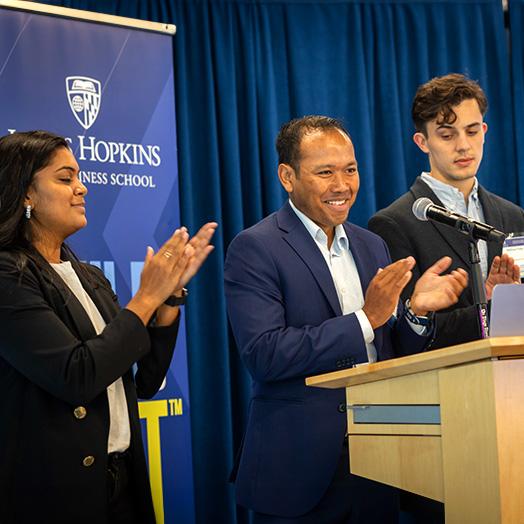
business of health
Emerging technologies and greater equity to shape the future of healthAmong the findings: Authentic reviews tend to contain more enthusiastic language and exclamation marks, often expressing a higher level of excitement, while fake reviews convey a more reserved and measured sentiment, focusing on the doctor’s professional attributes and expertise. In terms of writing style, real patients use casual and conversational language, often describing their personal experiences and feelings, while fraudsters use more formal language, with writing that is more structured and sentences that are more detailed and comprehensive.
These and other key differences, based on real-world reviews, come “in sharp contrast” to previous findings in the literature that were obtained using simulated data, note Agarwal and Gao.
Both say they are excited about the potential that large language models hold for improving the detection of fake physician reviews.
“Developing a method to detect such fraudulent behavior and pinpointing the key characteristics distinguishing fake reviews is the first step toward eliminating [the behavior],” says Gao. “And we believe that generative models such as GPT-3 offer considerable promise of automatic detection with high accuracy.
“The application of these techniques extends into many other sectors where consumers rely on reviews to make purchase and consumption decisions such as products, restaurants, and hotels.”