Can AI predict what consumers are willing to pay for goods and services? Carey research has important implications for policymakers and anti-trust authorities.
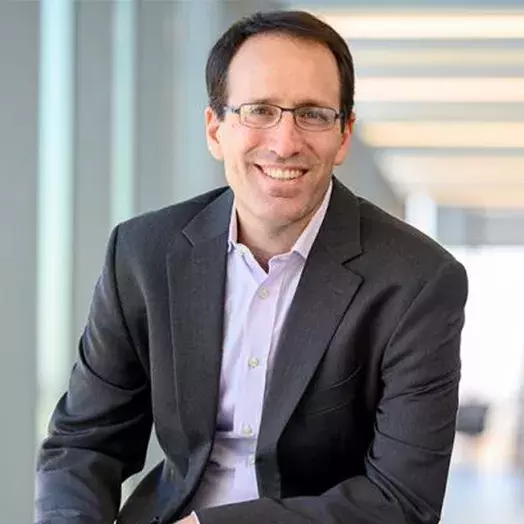
Is consumer profiling harmful for online shoppers?
Most online shoppers know that marketplace platforms collect consumer data that can be used to profile consumers and that the data can be shared with sellers.
“As economists, we are interested in better understanding how such data can be used to learn how much consumers are willing to pay for products, and how this knowledge can be used by sellers and platforms to set prices,” says Itay Fainmesser, an associate professor of economics at Johns Hopkins Carey Business School.
“There’s been this implicit assumption that marketplace platforms have so much data on our individual buying behavior that they can use AI to determine how much we are willing to pay for a particular item,” says Fainmesser. “But can they really?”
In a recent working paper, “Consumer Profiling via Information Design,” Fainmesser and colleagues Andrea Galeotti, of the London Business School, and Ruslan Momot, of the University of Michigan Ross School of Business, explored that question.
Their work has important implications for policymakers and anti-trust authorities given rising concerns “that prominent platforms can profile consumers accurately and use this information to manipulate market outcomes in ways that harm social welfare,” they note.
Learning from past purchases
In their research paper, Fainmesser and his colleagues investigate how a marketplace platform that initially lacks information about consumers’ valuations can learn it from their past purchases. The model does so by inferring valuations from consumers with similar observable characteristics.
“The platform might know that I am male and middle-aged, that I’m a university professor, as well as where I live and the types of things I buy. Then it looks at another buyer with the same characteristics and say, ‘these two are similar,’” explains Fainmesser. “But even if that’s done perfectly, that still doesn’t quite give them a sense of what I’m willing to pay. For that, you need to see past purchase decisions given a range of different prices.”
In the researchers’ model, the platform collects data on past purchases and can use these data to infer consumers' valuations, selectively disclosing information it has learned about consumers’ valuations to sellers. Importantly, the researchers note, that the marketplace platform doesn’t control the prices that are offered: Pricing is set by the sellers.
“We ask: How effective is information design for inducing sellers to price in a way that will enable learning consumers’ valuations — and what are the implications?” says Fainmesser.
What to Read Next
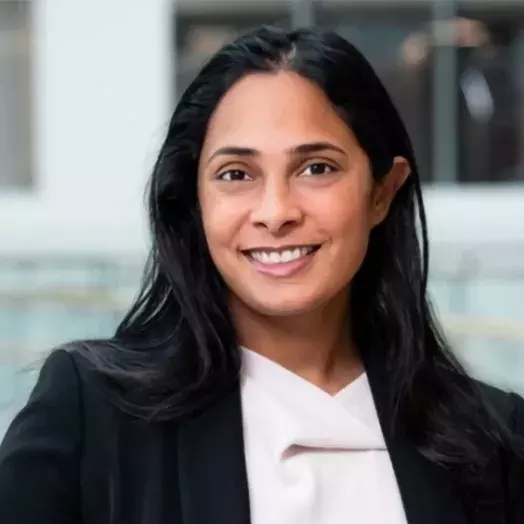
research
Overcoming bottlenecks in the transition to green technologyA reassuring threshold
What he and his colleagues found through their modeling should be reassuring to consumers and regulators alike.
Based on consumers’ past purchase information, platforms aren’t able to learn about very high valuations. “For example, a platform might wonder, can I find someone who is willing to pay $40,000 for a Honda Civic? But no seller is going to charge that, so there wouldn’t be enough data to support or refute that. This limits the ability of platforms to price discriminate with high valuations. There is a threshold above which the platform cannot accurately profile consumers,” Fainmesser says.
On the other hand, by carefully deciding what information to share with sellers, a marketplace platform can induce lower prices. As a result, there can be plenty of past purchase data to inform pricing for low valuation purchases, the researchers conclude.
“This is good for consumers because it allows sellers to target them with low prices,” Fainmesser says.
“Ultimately there’s a bigger lesson to be learned here,” he says. “The common assumption is that with enough data collection, platforms can learn everything. But that isn’t so simple. Our work shows one example of a common environment in which there’s a meaningful limit on what platforms can learn. This suggests that regulators may need to be more concerned about platforms which do more than matching sellers and buyers — those platforms that also have other ways to affect pricing.”