Academic and industry experts showcase the wide-ranging potential of a system that prioritizes solutions over predictions.
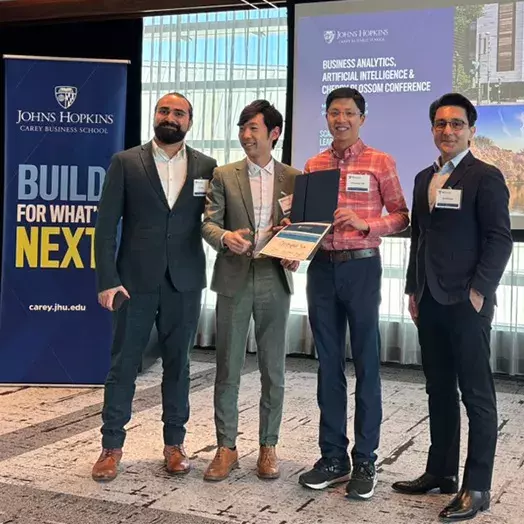
Carey AI conference examines the life-saving applications of decision-focused learning
If AI could not only make predictions but also optimize outcomes, it would change the way businesses, governments, and nonprofits gather data and make decisions. At the recent Johns Hopkins Carey Business School Business Analytics, Artificial Intelligence, and Cherry Blossom Conference, leading AI thinkers in academia and industry explored a powerful new way of thinking about and using AI—a framework called decision-focused learning.
Decision-focused learning is a departure from previous AI models, which gather enormous amounts of data in order to make predictions, which users then study to choose courses of action. Instead, DFL is less interested in perfect predictions and more concerned with robust outcomes. By integrating optimization problems into its learning mechanisms, DFL creates systems with vast potential to provide real-time solutions to complicated problems, even when they are riddled with unknowns.
“In the past, when we had uncertainty in optimization environments, for example when managing inventory, people would first run some prediction models and then they would optimize,” said Carey Professor of Practice Ali Ashragh, who organized the conference with colleagues Ali Fattahi and Yuexing Li, both Carey assistant professors.
“Nowadays,” Ashragh continued, “because of recent advances in AI and machine learning and the Big Data era, the concept is that instead of doing these two steps separately, we can put them together and look at them as one framework.”
Ashragh said he learned of the DFL concept in 2023, at the International Symposium on Forecasting, in a talk delivered by Georgia Institute of Technology Assistant Professor Kai Wang, who, at the time, was completing his Harvard PhD under the supervision of Milind Tambe. Tambe is the Gordon McKay Professor of Computer Science at Harvard University and Principal Scientist and Director for “AI for Social Good” at Google Deepmind.
Tambe and Wang were among the five high-powered speakers at the all-day event, which attracted about 75 attendees in academia and industry. Like others, they gave examples of how DFL is already transforming decision-making, and how its potential is likely to grow.
What to Read Next
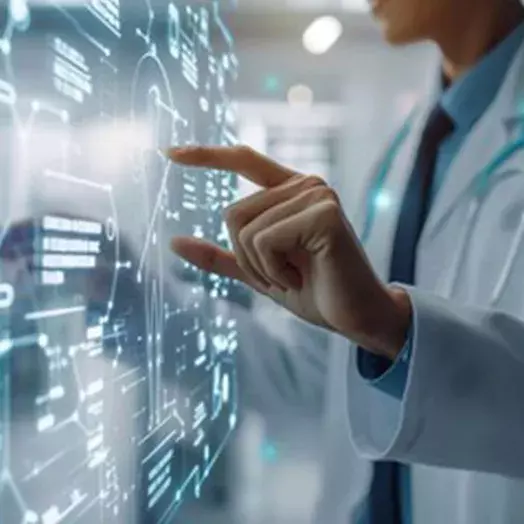
Faculty
AI for better health: Carey joins national effort to build workforce and capacityReal-world applications
Tambe said he’s particularly interested in using AI for social impact goals like improving health and public safety. He described how DFL allowed a maternal health nonprofit in India to encourage more mothers to stick with a program of automated telephone calls about nutrition and childcare.
Some 200,000 women participate in the program, he said, which delivers 141 messages from the time of registration through the first year of a child’s life. However, 30 percent to 40 percent of participants drop out, or listen only rarely, and therefore don’t benefit as much as they could.
Live phone calls explaining the value of listening to the messages can reverse this trend, but resources allow for just 1,000 such calls per month. The challenge for artificial intelligence was determining which mothers to contact.
AI, he said, could gather data about all the enrolled mothers, and attempt to predict which ones would stick with the messaging system.
DFL, he explained, focuses not on accurate predictions, but instead on optimal results, in this case the fewest dropouts, and integrates that optimization into its learning process. “The key observation is that maximizing learning accuracy is not the same as maximizing decision quality,” Tambe explained.
The results of the study, whose authors include Tambe and Wang and is considered the first real-world use of DFL, showed that 31 percent fewer women dropped out with the continued optimization of DFL, compared to the previous two-step process of first making predictions and then deciding which mothers to telephone.
Christopher Yeh, a fifth-year doctoral student at the California Institute of Technology, traveled across the country for the conference specifically to hear Tambe, and said he was impressed by the sophistication of the entire conference. Wang, he said, gave one of the best surveys of the state of the industry he’s ever heard. “It was very clear, and I got so much out of it,” he said. “It feels really nice to be part of this community.”
Sheldon White, a graduate of Johns Hopkins Whiting School of Engineering who now works for Roche Diagnostics, said he’s interested in how the concepts can help him with decisions about research and development investments. The goal, he said, is to use AI to “be flexible and agile, and minimize having situations where you’re not proactive in what you’re trying to do.”
Life- and resource-saving research
Also presenting at the conference were Pascal Van Hentenryck, the A. Russell Chandler III Chair Professor at the H. Milton Stewart School of Industrial and Systems Engineering at the Georgia Institute of Technology; Hamsa Bastani, associate professor at the University of Pennsylvania’s Wharton School; and Vahid Rostami, director of machine learning at the AI firm Pendulum Systems.
Rostami joined other speakers in noting the vast potential for systems that learn as they go and discussed the challenges and opportunities of using large language models to generate actionable strategies.
“A lot of decision-makers don’t even know what they want,” he said. “Sometimes they want to play with different variables. There is a lot of under-investigated potential in the current system to learn and maybe adapt to other problems.”
Van Hentenryck discussed using DFL to make real-time decisions about energy purchases and use, including when to charge and discharge batteries. “Energy generation and consumption changes fast, and reserves are changing fast as well,” he said. “Decision-focused learning is the next frontier.”
Bastani, co-director of the Wharton Healthcare Analytics Lab, provided another real-world example of how DFL can literally save lives, showing how decision-focused learning improved distribution of maternal and family health products across a sprawling health care system in Sierra Leone, despite the difficulties of predicting demand at each location.
The goal, she said, was not to predict demand, but to ensure that the correct products were available to anyone who needed them. “Classic machine learning says, ‘I want to predict well everywhere,’ but actually I only care about patients being turned away, so we focused on understocked facilities,” she said.
The result: a 19 percent increase in medication consumption overall, and a 44 percent increase in historically underserved facilities. “A lot more people are able to access their medicines,” she said. “This is so cheap. There’s no change in supply. They’re still using the same number of trucks and the same number of warehouses.”